Reimagine your project data to eliminate bias and maximise success
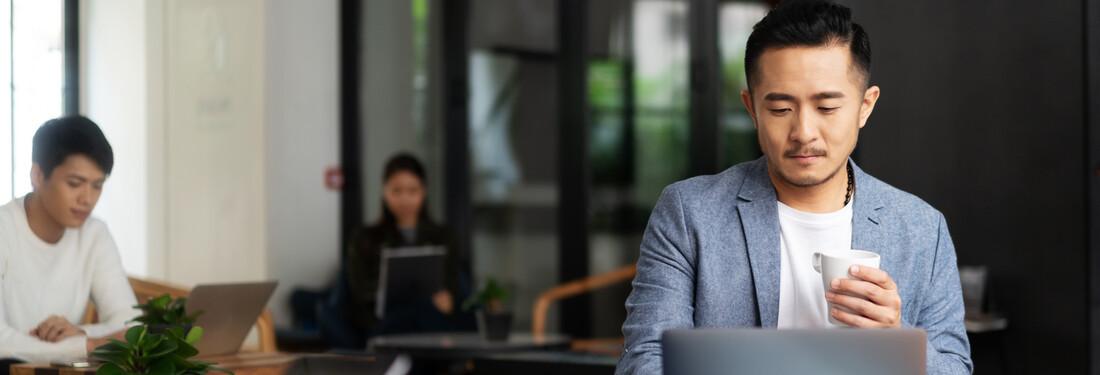
Whether we like it or not, data sits at the heart of everything we do in project management.
In the first of this series of articles on data and effective governance in project management, we learned that, as a profession, our success rates have hardly improved in the past 70 years. Greg Krawczyk ChPP of APM’s Governance Specific Interest Group attributed it to our vulnerability to bias and strategic misrepresentation during the project planning process.
But the good news is that both weaknesses, Krawczyk believes, can be overcome by harnessing the power of project data – specifically, reference class data that takes bottom-up project estimating to the next level.
In this article, we’ll look at which data Krawczyk recommends surfacing, how to collect it consistently and how PMOs can enable project professionals to eliminate the effects of optimism bias.
1. Understand reference class forecasting
You don’t have to be a data whizz to get on board with reference class forecasting. We won’t be reinventing the wheel here, we’ll simply provide a different perspective to help you identify your weakness to bias.
To set the scene, let’s start with a definition of reference class forecasting: “Reference class forecasting is a method of predicting the future by looking at similar past situations and their outcomes.”
Predicting the future? Sounds great. I asked Krawczyk to explain how it works.
“Think about your last project. Against your original estimates, did you deliver on time, or to cost or the expected benefits? What about all three? Like me, you probably know the answer. Oxford Global Projects research shows us that only 0.5% get it right across the board. Welcome to the 99.5% club.
“Your project estimates, whether comparative, parametric or bottom-up, are great at providing an inside view. That is to say, they help you estimate the known knowns. You’ll also identify some risks and build in some fat. Let’s call that allowing for known unknowns. But what about those pesky unknown unknowns? For those, reference class forecasting is a solution – crystal ball gazing, but with flair.
“So, how does it work? With reference class forecasts, you put yourself in the shoes of your predecessors. By looking at their original baselines, you can see how others modelled time, cost and benefit estimates. Then, with the project actuals collected, you can see the delta between the business case and the true delivery – that’s their optimism bias missing the unknown unknowns.”
2. Create structures that deliver clean and consistent data
A reference class data set is only as good as the quality and volume of data within it. So, what structures do we need to implement to consistently collect delta-defining data? Let’s hear from Krawczyk again to tell us how:
“I once attended a talk by a NASA cost estimator. He told a story of how, in 2000, Congress told NASA to sort out its estimating mess or risk losing funding. By 2015 that all changed. Now, NASA could estimate a project like Hubble with a high enough degree of accuracy that, to paraphrase, ‘If you told Congress back in 2000 how accurate we would be in 2015, they would have rolled around on the floor laughing’.”
So, how did NASA turn it around?
“Put simply, they completely standardised their classification and measurement techniques. They embarked on a transformation programme to improve how data points were modelled and therefore collected. Eventually, even the most sceptical project teams saw the light and staff couldn’t wait to ask which cost code their widget should go against.
“For those without planet-sized budgets, start by standardising your cost, schedule and benefit categories. From there, start capturing data – Microsoft Lists is a great free tool I use.”
3. Enable project teams to fight off the unknown unknowns
With the data collected, the final piece of the puzzle is to combine it, scale it and make it available to project teams to help them fight off their unknown unknowns. So how can organisations do this?
“The lesson I take from the NASA story is that the PMO has a critical part to play,” says Krawczyk. “The PMO collates, triages and disseminates the data, along with providing the tools, training and techniques that enable project professionals to level up their estimating capabilities.
“As the PMO collects more and more data, it’s all about modelling sets of projects together to create a clear picture of asset-specific estimating deltas.
“Then, unless project teams can argue they’re much better estimators than their peers, they’re armed with the insight to identify and overcome their own optimism bias and boost their chances of success. That’s because they now have the complete inside and outside view – the known knowns, unknown knowns and, thanks to reference class forecasting, the unknown unknowns.”
To wrap up…
When used alongside other techniques, reference class forecasting delivers a reliable way to boost your chances of project success. While the maths can be complicated, even a simple application should dramatically improve most (if not all) of your business case estimates.
But to guarantee the success of reference class forecasting, in the next article of this series, we’ll look at how organisations can apply effective governance structures to prevent those who would misrepresent the data in pursuit of their own selfish aims.
This article forms part of a series on data and effective governance in project management, led by the APM Governance SIG. Throughout 2023, the SIG will be driving forward our collective knowledge on this topic. To find out more about the SIG or get involved, click here or contact Greg Krawczyk directly.
0 comments
Log in to post a comment, or create an account if you don't have one already.