Artificial Intelligence: How Hong Kong uses 2,700 years of project data to shape today’s projects
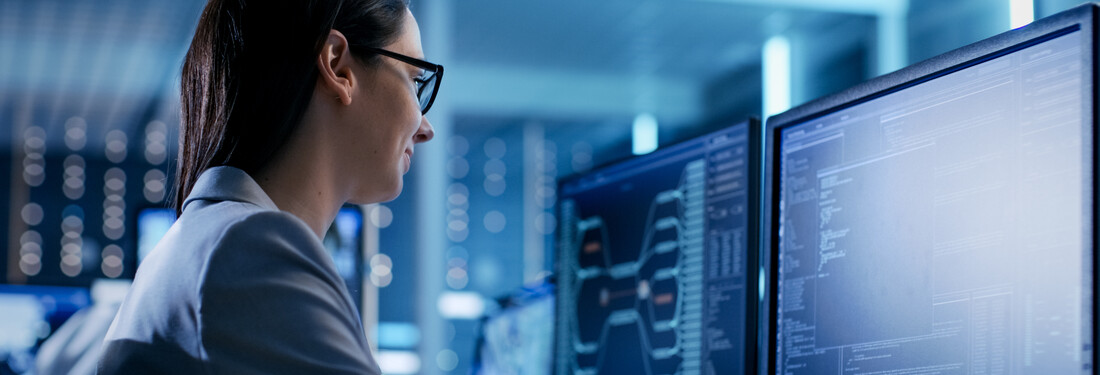
The Hong Kong Development Bureau’s Project Strategy and Governance Office wanted to use their extensive project cashflow records to create useful insights for delivering projects. With access to historical records of HKD 450bn and 2,700 years of construction projects, as well as a 42% increase in project spending from 2021 to 2031, the team wanted to make sure the projects were delivered as efficiently as possible. They wanted to be able to intervene early, rather than late, if a project was running awry.
Projects in Hong Kong are in the highly unusual position of underrunning project budgets more frequently then overrunning them. The average project is 15% cheaper than forecasted and only 10% of projects required additional funding beyond the approved project estimate. Whilst project underruns are better than blowing through budgets, it still brings problems: this means more money is held in contingency than required and there may be fewer projects delivered than was financially feasible across the portfolio.
With access to capital works projects data, Oxford Global Projects worked with the Hong Kong Development Bureau to develop an artificial intelligence (AI) tool that would use project cashflows over time as an early-warning system: the Project Supervision System (PSS). The tool measured annual cashflow as a percentage of the (1) final project cost and (2) the original planned cost. The AI found that project performance varied based on whether the project was a building or non-building project. Building project performance was dependent on whether the height was 150m or less and non-building projects varied by planned duration, with six years as the turning point.
The PSS assigned early warning flags based on cashflow performance:
This raised awareness of projects that were at risk of significant overspending, underspending, or schedule delays. If left unchecked, the amber and red projects could become drags on Hong Kong's ambitious development portfolio. The AI was up to 20 times more accurate than assigning random flags for project performance. For example, a red flag over spend was accurately forecast by the AI 69% of the time, whilst random assignment would anticipate a red flag cost overrun 3% of the time.
The Project Strategy and Governance Office gave more support and leadership intervention to projects that were flagged amber or red for cost or schedule performance. Amber projects were reviewed by the Heads of Departments who took recovery actions and red projects were dealt with at regular senior leadership meetings chaired by the Permanent Secretary for Development (Works).
The next stage of this AI research project was to predict final project costs using just
- the percentage of progress the project had achieved
- the planned project duration at final investment decision
- the cashflow spent thus far as percentage of the approved budget.
This required a more advanced form of AI – a deep neural network. This AI had an error of only ±8% on forecasted portfolio costs. This meets international best practice standard recognised by AACE International.
What does this look like in practice?
An example is a project with an approved project cost estimate of HKD 5.2bn with completion within 3.5 years. It has completed its first year of construction.
The cashflow information showed that the project had spent 1% of its cash but it was nearly 29% complete when measured against the baseline duration. The AI assigned this project a red flag because it’s been spending the budget far slower than the AI anticipates based on the typical spending pattern of capital works projects in Hong Kong. The project team forecasts that this will reverse in later years, but the AI raises this for further investigation by the Permanent Secretary and senior management team.[4]
Whilst this project has a budget of HKD 4.0bn, the AI forecasts that a cost of HKD 5.1bn is more likely. This information enables early intervention and an appreciation of what level of investment is proportionate to remedy the project. The senior leadership team would likely evaluate the rigour underlying the forecasted acceleration and whether it is feasible.
My favourite project heuristic is to get projects right from the start because the earlier risks and issues are addressed, the cheaper and easier it is to put things right. The AI intervention allowed project leaders to prioritise their efforts more effectively and give attention to projects that needed help. Since putting these AI tools in place, the Development Bureau of Hong Kong has optimized project budget allocations across the department by 10%. This has resulted in an annual saving of nearly EUR 1.5bn across 500+ projects. Learn more about this case study by reading AI in Action here. AI is globally recognised as a tool for shaping and improving the performance of projects. It is vital that project leaders embrace technological tools to deliver projects better.
You may also be interested in:
[1] For <5th or >95th percentile
[2] For 5th–30th and 70th–95th percentile
[3] 30th–70th percentile
[4] Flyvbjerg, Bent, Budzier, Alexander, Chun-kit, Ricky LAU, Agard, Karlene, Leed, Andreas (2022) AI in Action: How the Hong Kong Development Bureau Built the PSS, an Early-Warning-Sign System for Public Works Projects. Available at https://www.psgo.gov.hk/assets/pdf/AI-IN-ACTION-Whitepaper.pdf.
1 comments
Log in to post a comment, or create an account if you don't have one already.
Interesting case @karlene. The variables used seem substantively the same as those used by Earned Value. Do you have an assessment of how the Ai system compared to the conventional EVM method? Whilst the two cases in the whitepaper show the actual cost graph, they do not show the associated PV and EV, so I wondered whether the HK projects used this execution measurement tool. And if there are differences do they relate to known weaknesses in the EVM method, or are they new insights? And if new have they been fed back to the APM SIG that oversees the APM EVM Handbook?